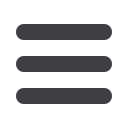
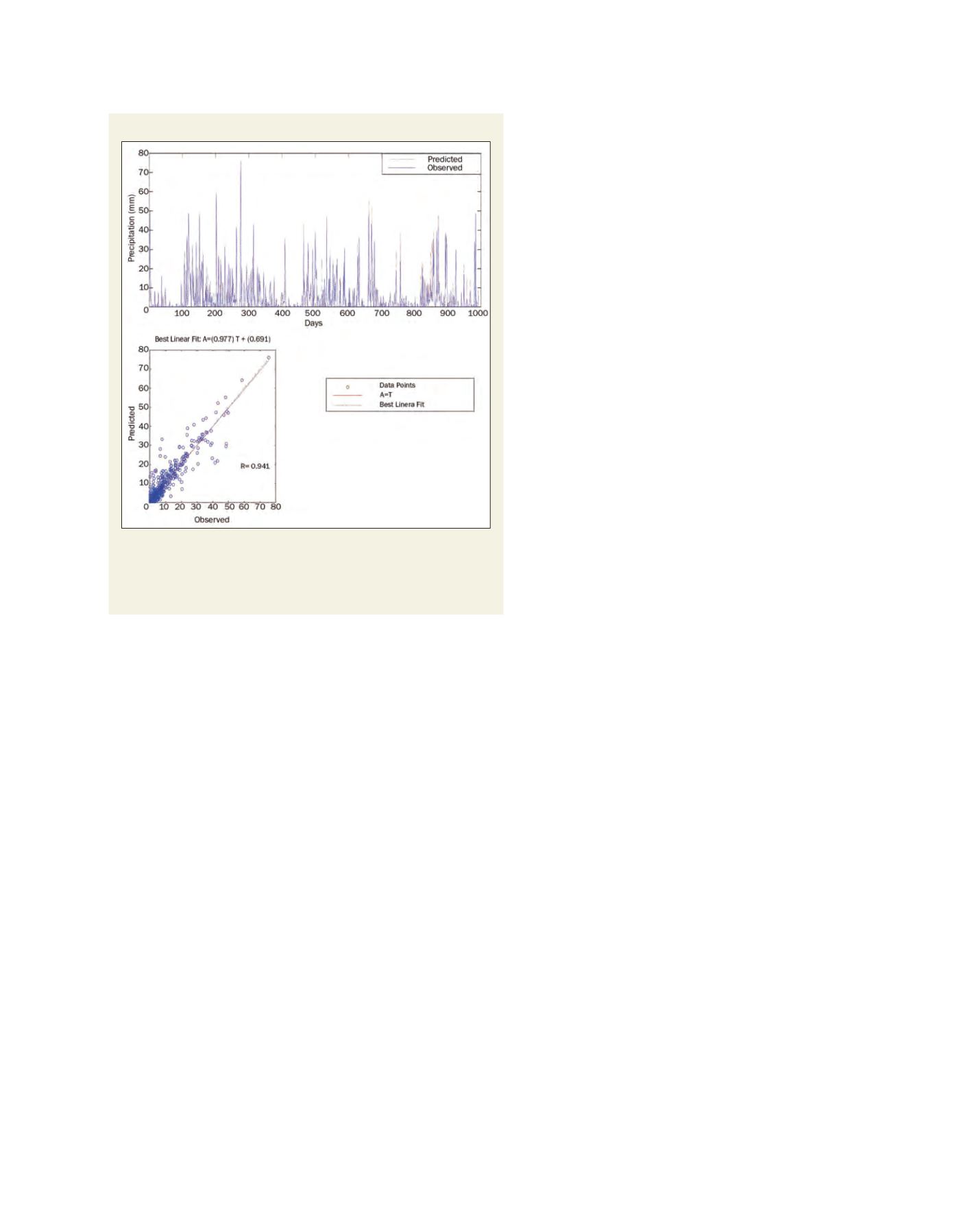
[
] 242
A
daptation
and
M
itigation
S
trategies
Comparison between observed and predicted precipitation
Time series of observed and artificial neural network reconstructed
precipitation for 30 per cent of data randomly degraded (top) and scatter
plot of reconstructed against observed precipitation for 30 per cent
degraded data (bottom)
Source: Caribbean Institute for Meteorology and Hydrology (CIMH)
be appropriate to predict climate change, but a combined approach
where regional models are also statistically downscaled would be
valuable. Additionally, islands that are small, irregular land masses
would benefit greatly from high resolution modelling, as processes
such as land/sea breezes, which are a result of the different thermal
characteristics of land and ocean, cannot be captured by a GCM.
The type of climate information required may also influence
whether or not high resolution modelling is desirable. Certain
surface variables, such as surface temperature or precipitation, are
more likely to be significantly improved by using high-resolution
data as opposed to atmosphere variables such as 500 hectopascal
heights. One may have a case where a low resolution model gives
fairly accurate mean rainfall, however capturing extreme precipita-
tion events usually requires high resolution models.
In recent years, there has been an increase in use of RCMs through-
out the Caribbean. These models use dynamical downscaling and
provide more accurate representations of physical processes. There are
a number of Caribbean institutions – Climate Studies Group Mona
(CSGM), University of the West Indies, Instituto de Meteorologia de
la Republic de Cuba and the Caribbean Community Climate Change
Centre –that, through their collaborative efforts, have produced
regional climate change projections that can be used by researchers
and policy makers to assess potential impacts and develop adaptation
plans. Extensive work is being done to ensure these products will
be readily accessible and available to the wider community in addi-
tion to universities and research centres. CSGM also uses statistical
downscaling to downscale global projections to specific observa-
tional stations. Statistical downscaling of temperature
and precipitation from global models to station sites
in Barbados, Jamaica and Trinidad was undertaken in
an Assessments of Impacts and Adaptations to Climate
Change in Multiple Regions and Sector Small Island
States SIS06 project. Information from these climate
scenarios would be useful to policy makers for charting
adaptation and mitigation strategies for the region.
CIMH has investigated the plausibility of using an
artificial neural network (ANN) to reconstruct missing
daily precipitation data for a rainfall station in Guyana.
This functionality was tested by asking ANN to predict
data at a station using incomplete data as well as data
from nearby stations. Comparisons of the predicted
data to actual measured data at the site showed good
correlation, even though the data given to ANN had
30 per cent of its values randomly removed. Further
ongoing studies are being conducted to assess the effec-
tiveness of the ANN technique for longer time series. If
successful, this would support statistical downscaling
of climate models and climate change impact studies in
the Caribbean region.
Future work at CIMH
Future climate-oriented work at CIMH will focus
on investigating long-term climate variability with
the intention of improving predictions and enhanc-
ing understanding of climate change in the region.
Research will be conducted using mesoscale climate
models such as the weather research and forecasting
model to obtain information about the variability of
climate in the region and to determine the medium-
to long-term variability of weather systems affecting
Caribbean climate.
Additional research will use mesoscale climate models
to examine the effects of Saharan dust on Caribbean
climate. Long-term monitoring studies in the Caribbean
region have shown large interannual increases in atmos-
pheric dust concentrations. Atmospheric dust can
influence the Earth’s radiative budget directly, by scat-
tering solar radiation in the atmosphere, and indirectly,
by changing cloud condensation nuclei concentrations.
Dust has also been linked to reduced precipitation
in the Caribbean and to changes in seasonal rainfall
patterns.
2
It may also be a major source of tropical
hurricane suppression in the Northern Tropical Atlantic
due to factors such as cooling of sea surface tempera-
tures in the main hurricane development region
3
and
the Saharan Air Layer.
4
A key regional initiative that would be of great benefit
to any mitigation and adaptation strategy would be an
objective intercomparability and assessment study,
which would enhance understanding of the range and
causes of uncertainties in climate model outputs and the
model biases for the Caribbean region. It would also be
useful in showing how model predictions may change
according to different scenarios. Information from all
these model simulations and intercomparisons would
be useful for adaptation and mitigation initiatives.